By Obim Okongwu
Introduction
Artificial intelligence (AI) has recently piqued the world’s curiosity. While the trigger for all this attention was the capabilities of generative AI and large language models along with chatbots such as ChatGPT, the promise of AI is clear.
From its ability to provide insights to support better health care and guide medical decisions, to improving customer experience, to refining underwriting and pricing – the benefits of AI seem endless. AI also helps us understand the impact of climate change, helps financial advisors tailor solutions and services, supports better research and development for scientific discoveries, writes software code and summarizes reports. There are views that AI has the potential to radically transform our economy, our society and humanity.
These benefits are made possible due to the massive data available for the AI algorithms, which continue to grow exponentially due to digitization. In addition, costs of both data storage and computing power continue to drop, while more sophisticated algorithms continue to be created.
However, despite the benefits, AI presents the situational “double-edge sword.” Concerns have been raised on the trustworthiness of AI. One of those concerns is the potential for subtle bias being picked up by AI algorithms from data they are trained on and then magnified significantly. AI algorithms can detect incredibly complex patterns from massive data. The algorithms can also be quite complex leading to their sometimes black-box nature making it difficult to explain and to ascertain robustness. Privacy, intellectual property and legal issues have been highlighted with generative AI.
The risks of AI
Traditional model risks not only persist with AI but could also be exacerbated. There are other risks that could emanate from the adoption and use of AI illustrated in Figure 1 below.
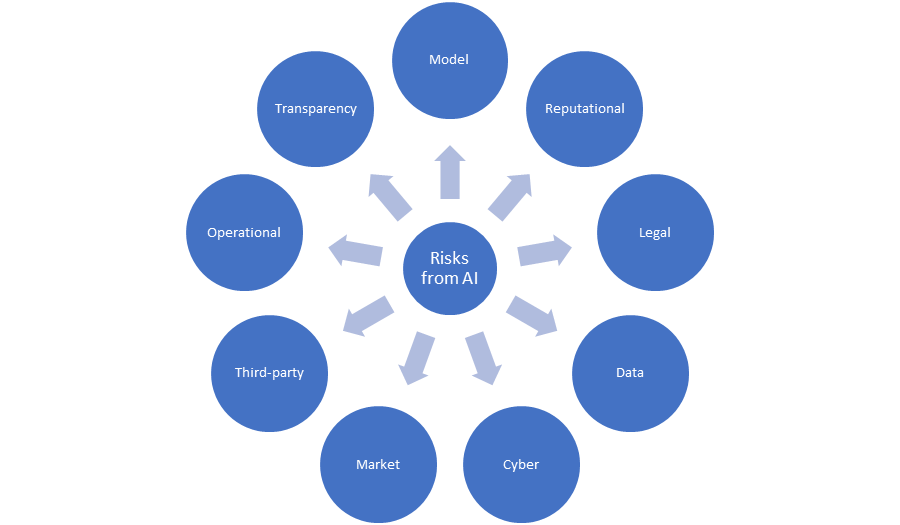
The positive aspect of AI lies in its ability to learn patterns, even astonishing ones, from significant data size. Problems arise if the AI model is overtrained, if data in the real world (or production) does not align with training data and patterns learned or if the algorithm learns something from the data that developers are unaware of. Therefore, if any or some of those problems occur, there is the increased potential for incorrect results, more so, if the model is used for purposes that it was not trained for.
The complexity of some AI algorithms makes it difficult to understand how results are produced or even explain those results. There are also greater challenges from self-learning or dynamic models that learn while in production.
The sheer size of data used by AI, which keeps growing, as well as data variety and velocity, increases the challenge to govern data and maintain data integrity and quality. With AI systems being data-driven and more interconnected, they may become more vulnerable to hacking and cyberattacks. Data and model poisoning attack, by adding special samples to training data sets or embedding malware in open-source code, can occur to mislead AI systems during operations or create vulnerabilities.
Besides hacking, which could expose private data, the sheer desire to leverage more and more data for insights and benefits could lead to the collection and use of private data or data without consent. AI has the capability to unmask anonymized data. In addition, AI model outcomes may leak sensitive data directly or by inference. AI’s power of detecting astonishing patterns enables it to learn subtle biases and magnifies them, leading to unintended consequences. There’s the argument to exclude protected variables such as race and gender but those may be inferred by the algorithms. Allegations of possible discrimination from unfair outputs as well as from privacy breaches, could lead to reputational damage or legal issues for an institution.
Without large teams to build and validate AI models, there is likelihood of reliance on third parties. This is particular for smaller-sized institutions. Besides actual models, there could be increased reliance on third party data in order to reap AI benefits. The use of open-source code and libraries is inevitable. All these increase third-party risks and potential concentration risks. Another inevitability is the increased use of and reliance on automation and potential autonomous AI. Failure of automated processes or autonomous AI can have significant consequences for a downstream or related process, thus, the operational risk implications.
Regulations for AI
While there has been a recent increase in calls for AI regulations, prompted by popularity and concerns with large language models, policy makers and regulators around the world have been carrying out various activities over the past couple of years.
Global regulations
Policy makers and regulators have been considering how to encourage the economic and social benefits of AI while managing its risks, challenges and downsides such as concerns regarding systemic issues and harm to their citizens. To achieve that balance, many began with issuing discussion and public consultation papers, principles and reports.
“Though the few AI specific policies and regulations are in draft form, it is worthwhile to note that AI policies and regulations are preceded by AI principles.”
– Obim Okongwu
Policies and regulations are normally guided by the jurisdiction’s AI principles and input from different stakeholders. While approaches being taken by different jurisdictions could differ, some more stringent than others or some principles-based vs. prescriptive, indications are that they will all be risk based.
The EU is expected to finalize the first ever comprehensive legal framework on AI this year. Its AI Act is being seen as the strictest and will have global impact due to large organizations being multinational, AI system developers in other countries will naturally market to the EU due to its significance with almost 450 million population.
The EU AI Act regulates AI based on risk categories – unacceptable, high and low/minimal. Specifically, algorithms used for the risk assessment and pricing of health and life insurance are considered high-risk and must meet more stringent regulatory requirements.
Canadian regulations
In Canada, upcoming policies and regulations that will impact the financial sector are the Office of the Superintendent of Financial Institutions’ (OSFI) “Enterprise-Wide Model Risk Management Guideline” (E-23) and the Artificial Intelligence and Data Act (AIDA) within Bill C-27.
OSFI’s E-23
Though E-23 is yet to published, OSFI issued a letter in May 2022 on aspects being considered for all models, including AI models. Some models are developed with AI algorithms.
With data becoming more available, increasing in size and used for modelling, it was a key point of consideration, from lineage, provenance and quality, to governance. The rigour of developers, validators and model approvers to ensure the model put in production is robust was also going to be considered. There was also recognition that unfair bias in data can be magnified by AI models with potential reputational implications.
While documentation is important for business continuity, the level of documentation commensurate with model risk was also going to be considered. It recognized that institutions may have existing governance structures, therefore, the extent to which structures could be enhanced was to be considered. The presence of different dimensions and levels of explainability was recognized.
In all of these, expected guidance was going to be commensurate with risk. In addition, proportionality was to be covered.
Bill C-27 – AIDA
AIDA was tabled in June 2022 as part of Bill C-27, the Digital Charter Implementation Act – 2022. A companion document was released in March 2023.
“AIDA recognizes that Canada needs to be a thriving advanced data economy, thus, requires a framework that inspires citizen trust, encourages responsible innovation and remains interoperable with international markets.”
– Obim Okongwu
It addresses two types of adverse impacts, (1) harm to individuals (physical or psychological harm, damage to property or economic loss); (2) systemic bias in AI systems in a commercial context with unjustified and adverse differential impact based on any of prohibited grounds reflected in the Canadian Human Rights Act.
Like the EU AI Act, it will be risk based though focused on high impact systems. Examples of high impact systems are those with potential for risk of harm, the severity of potential harm, scale of use and where it is not possible to opt out from the system.
There will be expectations for businesses that design or develop a high impact system; businesses that make them available for use; and businesses that manage the operations of an AI system. The expectations will cover identification, mitigation, documentation, monitoring and disclosure of the risks; with those guided by a set of AIDA principles. For noncompliance, there could be monetary penalties or prosecutable regulatory offences.
Principles for responsible AI
Principles to mitigate those risks and help guide the responsible adoption and use of AI have been highlighted by different policy makers, regulators and organizations in various countries. The principles highlighted by Canadian policy makers and regulators align with those presented in other jurisdictions. Figure 2 below presents various principles, global vs. Canadian.
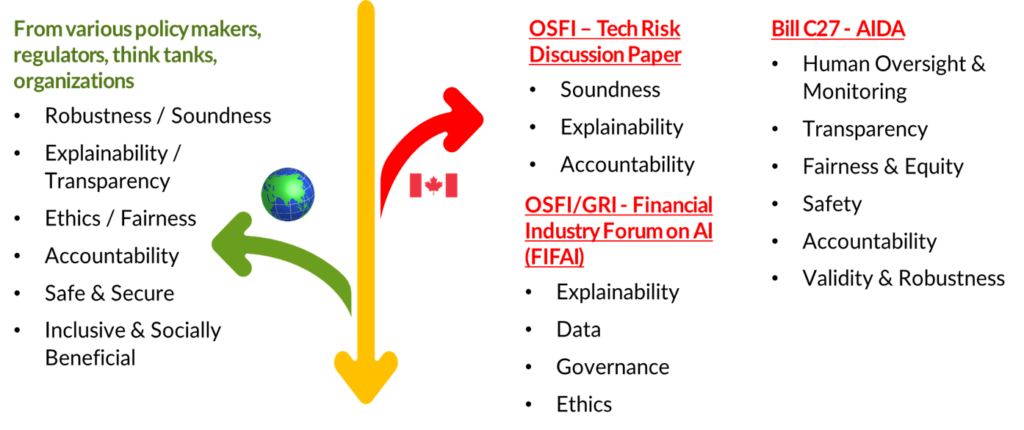
In Canada, three principles (soundness, accountability, explainability) were presented by OSFI in its 2020 technology risk discussion paper. These were followed by four principles (explainability, data, governance, ethics) that arose from the Financial Industry Forum on Artificial Intelligence (FIFAI) organized by OSFI and the Global Risk Institute. The FIFAI report, “A Canadian Perspective on Responsible AI,” was published in April 2023. The companion document of AIDA presents six AI principles (human oversight and monitoring, transparency, fairness and equity, safety, accountability, validity and robustness).
AI principles tend to guide policies and regulations, the AIDA companion document states that expectations will be guided by the outlined set of principles. Institutions could leverage the principles to develop or enhance existing model risk management frameworks. A few notes on some principles are presented below.
- Robustness or soundness ensures that the model is reliable and performs as expected while in production in various situations, even edge cases[1].
- Explainability and transparency encompass understanding the data used to train the model, the inner workings of the model and the output. They also encompass proactively disclosing applicable and appropriate details to various stakeholders and in the “language” the applicable stakeholder is able to understand.
- Ethics ensures model outputs are fair and without negative biases. It also encompasses privacy and consent.
- Data encompasses quality, provenance, completeness, privacy and consent considerations for ethical use, all important elements enabled by sound data governance.
- Accountability and governance ensure the right people or teams are involved and responsible for every step of the model lifecycle as well as the presence of policies and governance structures, and thus, ensure all principles for responsible AI adoption and use.
- Safe and secure means that AI should also be safe for individuals and secure for individuals and organizations considering the exposure to cyber threat due to more data leveraged and digitalization.
- AI needs to benefit everyone, contributing to the overall growth and prosperity for our society.
Looking ahead at the future of AI
It is inevitable that innovation and sophistication in AI will continue, with organizations needing to leverage it in order to remain competitive. With potential for risks to be exacerbated with such advancement and use, calls for AI regulation have increased in recent months from renowned AI researchers and leaders of prominent AI companies.
Regulators and policy makers in various jurisdictions will continue to act to ensure the soundness of their institutions and protect their citizens.
How has AI been transforming your workspace and field? Share your thoughts below or contact us at SeeingBeyondRisk@cia-ica.ca and consider drafting an article for the Seeing Beyond Risk blog.
[1] Edge cases are rare occurrences, probable cases with low probability of occurrence.